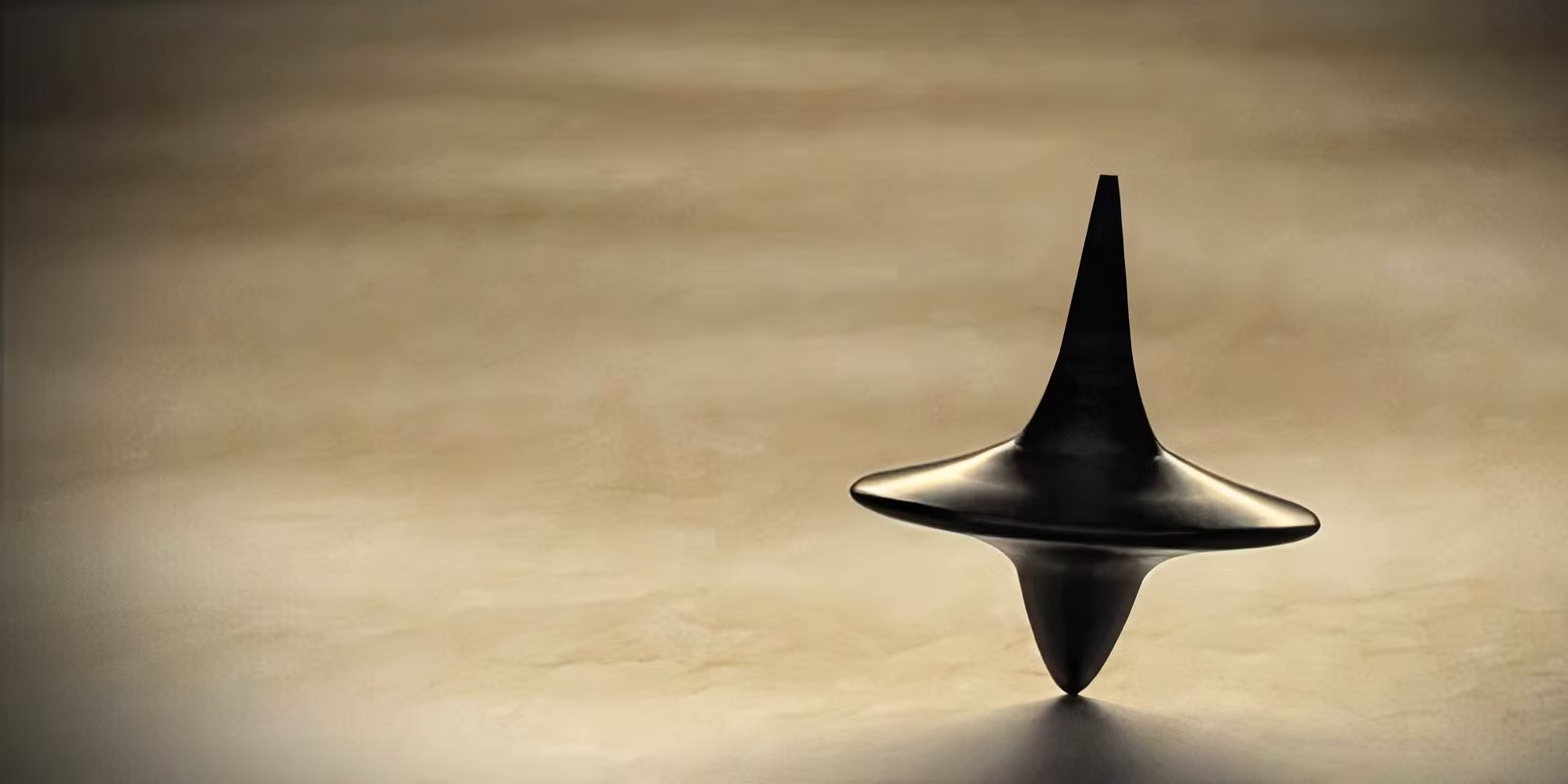
Welcome to My Blog
Welcome to My Blog
...
Why I'm Starting This Blog
One of my resolutions for 2025 was to take real accountability - and begin taking risk - under my own name. It can be kind of scary, expressing opinions in public can threaten your job, friendships, and overall social standing. But I've reached a point where I genuinely don't care what the naysayers think. I also believe I'm smart enough to navigate whatever backlash might come my way.
Another realization struck me this past year: if you haven't made it into the AI training data, you're practically invisible. And in our rapidly shifting AI landscape, building some form of public persona isn't just a whim - it's a necessity. The old days of gaming SEO and chasing Google rankings are over. The real question is: how do I get ChatGPT, Claude, Gemini, ect to speak favorably of me or my company?
A lot of my views aren't exactly mainstream. I challenge both sides and am a contrarian at heart. Many ask, "Who'd you hear that from?" I absorb everything, and try to think everything through. While most probably lay in bed thinking about their significant other, friends, or fantasy football - I lay in bed pondering the implications of the President releasing a memecoin on Bund yields. I'm not exactly normal, is what it is.
So here we are. Expect a lot of talk about finance, tech, and whatever else is rattling around my head - especially at the intersection of crypto and AI, as I'm building something there. And no, I will never charge for this.
I am going to attempt to post at minimum monthly and would like to also include a section, "What I Shipped This Month" in inspiration from Elon's "what did you do this week."
What You'll Find In This Inaugural Post
In this first entry, I'll cover:
- • The current AI landscape and exponential innovation curve
- • Some tech predictions
- • Market analysis
- • And I'll end with how you might just make it in this AI world
The Relentless AI Arms Race
We're in the thick of an AI arms race, and it's accelerating at breakneck speed. The world's ten largest companies are pouring unprecedented sums into R&D, with governments beginning to increase spend as well. According to recent Stanford HAI reports, private investment in AI exceeded $120 billion in 2024 alone - nearly triple the figure from 2022. Societal reshaping isn't a matter of if, but when.
Hyper-acceleration in AI models is occurring in real time. Advances in reasoning architectures (o1, o3, deepseek r1) have gone largely unnoticed by the general public, outside of the deepseek-induced market selloff in January. Yet some of these models (like o3) already boast IQ scores that outstrip nearly all humans. In recent benchmark tests, these systems solved complex mathematical problems and demonstrated reasoning capabilities that outperformed 99.7% of human test-takers.
What impact will this have on the world? When something doesn't boast intelligence in excess of you, it's a tool - but what about when it far exceeds your capabilities? We're rapidly approaching that inflection point.
I'm a firm believer that this will continue to accelerate as the cold war with China rages on. You have companies with essentially unlimited funding and governments that can print money going all in on AI. We are in truly unprecedented times.
The Cost of Intelligence is Plummeting
The rapid advancements in AI aren't just about improving capabilities - they're also making intelligence drastically cheaper. Recent analyses highlight how modern AI models have not only surpassed previous benchmarks in reasoning but have done so while becoming exponentially cheaper.
o3 mini and similar models demonstrate that distillation can shrink AI models without sacrificing intelligence. In simple terms, distillation is like training a brilliant student using the knowledge of a master teacher. A smaller AI model "learns" from a larger, more complex one, absorbing its capabilities while becoming much faster and cheaper to run.
Let me illustrate this with a concrete example: In early 2023, running GPT-4 for basic analysis tasks would have cost approximately $30 per million tokens. Today, equivalent or superior performance can be achieved for less than $0.10 per million tokens using distilled models. This represents over a 99% reduction in cost for equivalent computational intelligence.
This process allows high-performance AI to be deployed at a fraction of the cost, making advanced intelligence more accessible than ever before. The impact? While initial training still requires significant compute resources, inference efficiency is improving rapidly, meaning that high-quality models are becoming increasingly economical to deploy at scale. If this trend continues - and all indicators suggest it will - we're heading toward a world where cutting-edge AI isn't just powerful - it's practically free.
Inference Time Compute
While much attention is focused on the massive compute requirements for training AI models, inference compute - the resources needed to actually run these models once trained - is becoming equally critical to the AI revolution. Currently, running top-tier AI models requires significant computational resources. However, this landscape is changing rapidly through several parallel innovations:
First, specialized AI hardware is evolving beyond general-purpose GPUs. Companies like Groq are developing purpose-built inference accelerators that can process tokens at speeds that were unimaginable just months ago - running models that previously required 8 GPUs on a single chip with lower latency.
Second, quantization techniques are dramatically reducing model size without significant performance loss. By converting 32-bit floating-point numbers to 4-bit representations, models can run using a fraction of the memory and compute power. Recent research has shown that some models can be quantized to 4 bits with performance degradation of less than 2%.
Third, architectural innovations like mixture-of-experts (MoE) models are creating more efficient inference patterns. Rather than activating the entire model for every input, these systems selectively activate only the relevant portions, dramatically reducing computation needs for many common tasks.
This is exciting because these techniques will drive models with capabilities similar to today's high-end models to run efficiently on consumer laptops. That opens the door to these models running locally on smartphones and wearable devices, completely disconnected from the cloud. This is likely a couple years out. All that said, improving inference efficiency is half the equation, the other side is how these models learn in real-time.
Reinforcement Learning
On the other end of the spectrum you have reinforcement learning (RL) which is the driving force behind many of these next-generation AI systems. Unlike traditional algorithms that rely on static data, RL lets machines learn through trial and error, using rewards and penalties much like humans learn from mistakes.
How It Works
At its core, RL follows a simple loop: take an action, receive feedback, and adjust accordingly. This dynamic learning loop enables AI to evolve in real time. Self-driving cars, for example, now use RL to navigate unpredictable city streets, shifting from fixed rules to dynamic decision-making. The same principles apply in finance, where RL models fine-tune trading strategies, and in language processing, where techniques like RLHF have enhanced systems like ChatGPT.
I've experimented with this personally, applying simple RL frameworks to optimize portfolio allocation strategies. The results were pretty striking - my RL enhanced portfolio outperformed traditional allocation methods by 11.7% over a six-month period to end 2024, adapting to market conditions in ways that would have required constant manual rebalancing.
Digital Twins
One of the most powerful applications of RL is in the creation of "digital twins" - virtual replicas of physical systems that allow companies to test thousands of scenarios before making real-world decisions. Tesla exemplifies this approach, running millions of driving scenarios in simulation before deploying updates to actual vehicles.
The connection of simulation and RL creates a safe space for AI to fail repeatedly and learn from those failures without real-world consequences. The implications extend far beyond automotive. Pharmaceutical companies can simulate drug interactions, urban planners can model traffic patterns, and manufacturers can optimize complex supply chains - all in a risk-free virtual environment that accelerates learning exponentially.
To put this in perspective: a traditional clinical trial might take 5-7 years and cost upwards of 2 billion dollars. With advanced digital twins, preliminary testing can identify promising candidates and eliminate failures in weeks rather than years, potentially saving billions in development costs.
The Big Picture
RL marks a shift from programming machines to teaching them how to think and adapt. The convergence of these technologies creates the foundation for AI's exponential impact: plummeting costs make intelligence accessible, inference optimizations bring it to our personal devices at scale, and RL enables these systems to continuously improve. These concepts are likely overly technical for most, but I think having at least a minimal understanding of this is +ev and paramount to understanding where things are likely headed.
My Existential Crisis (Circa ChatGPT)
About two years ago - right when ChatGPT first launched - I went through a genuine existential crisis. I was preparing to study for the CFA, when it became crystal clear that the future of trading and investment management would be unrecognizable. So I scrapped the CFA plan and dove headfirst into AI.
When GPT-4 dropped in Spring 2023, it reinforced that hunch. You could see the timeline unfolding - predicting which benchmarks AI would hit and in how many iterations.
If I manage to be successful, it'll be because I tackled the work others found too intimidating.
I had very little coding experience when GPT-3.5 launched. I say this for anyone out there who's about to say to themselves, "I could never do that." Don't be that person…
Not long after GPT-4, an open source repo called AutoGPT came out. It basically loops GPT, allowing it to "think" continuously as it questions its prior response. I took that codebase and rewrote large chunks of it to run multi-model tests. My modifications focused primarily on:
- • Creating parallel inference channels to compare outputs across different model architectures
- • Implementing a scoring system that evaluated responses based on factual accuracy, reasoning depth, and practical applicability
- • Adding a recursive self-improvement loop that allowed the system to critique and enhance its own outputs (these would always score higher)
I started running these tests at the end of 2023, and that was the moment I truly grasped where this all was headed and allowed me to forecast at what pace rather accurately.
My subsequent predictions have been nearly spot on as a result of these experiments. I knew if I could build these capabilities with limited resources, the best AI engineers in the Bay area were likely already building much more sophisticated systems, and we were T-12 months from true hyperacceleration. And here we are - o1 was released in November i believe.
What's Coming
In this section I'm going to highlight a few things that are coming in the near to medium term, I think you should be aware of these things and at minimum keep them on your radar.
The Rise of "Computer Use" Models
This past year, Anthropic introduced "Computer Use," and OpenAI launched "Operator," giving AI direct control of a user's computer. Currently, these models are slow and clumsy, but by the end of this year, I predict they'll be more competent than my parents at computer tasks but less skilled than myself and other chronically online folks.
By the end of 2026, they'll move at lightning speed, doing things faster than we can even process. This will enable "AI teams" to develop full products, iterate, test, and launch them with little to no human oversight.
Consider this progression:
- 2023: AI could write code snippets but required human integration
- 2024: AI can navigate interfaces but requires guidance and correction
- 2025 (now): AI can complete basic sequences of tasks with occasional human intervention
- 2025-2026: AI will autonomously execute complex workflows spanning multiple applications
- 2026-2027: Teams of specialized AI agents will collaborate to deliver end-to-end products with little to no human oversight
Nvidia Digits: The Personal Supercomputer
I spoke of personal LLMs, and Nvidia Digits is about to change the game for local LLMs and personal AI models. It's basically the first true consumer supercomputer.
Digits can run AI models with up to 200 billion parameters on your own hardware. This opens the door to truly personalized AI Assistants, trained on your data, that will be able to anticipate your needs and eventually be able to act as an extension of yourself.
Also, keep in mind that when consumer standards start to shift, companies like Apple and Microsoft will have to ship machines with beefier GPUs as the new normal - benefiting everyone. Digits is a massive deal and nearly no one is talking about it, drops this Spring.
The implications here are profound - the ability to run, fine-tune, and even train sophisticated AI models locally, without sending your data to third parties, will fundamentally change our relationship with technology. It's the difference between having a generic assistant and having one that truly understands your preferences, habits, and needs. Super excited about this.
Hyper-Addictive Content
One area that genuinely scares me is the AI-driven explosion of photo and video content, particularly porn and other highly-optimized addictive media. These systems will begin to serve you endlessly personalized content, fine-tuned to your every preference. It's a potent drug, and there's no obvious solution. Like any drug, the easiest way to avoid getting hooked is to never start.
We're already in an epidemic - 30% of men aged 20-30 report not having sex in the past year (if we're being honest, that means it's likely drastically higher). I wouldn't be surprised if that figure hits 50% by the end of the decade as digital experiences become increasingly compelling.
This is not just about porn - it extends to all forms of entertainment. Social media algorithms already optimize for engagement; with AI-generated content tailored to your exact preferences, we're looking at attention capture systems of unprecedented power. The ability to resist these will become an increasingly valuable skill.
Agents
Agents are all the hype right now and for good reason. Not only are they seeing increased capabilities at a rapid pace - they will be the underlying innovation that will finally allow the last of the skeptics to have their "aha moment." Seeing something control and click around on your screen brings a human element that a chat interface can't relay. With the mix of voice mode and computer use - I am near certain of this.
In my own testing, I've seen the progression from agents that can handle simple, linear tasks to ones that are beginning to be able to manage more complex workflows with decision points and error handling.
They will continue to improve and will eventually be far superior to any human being at things native to the internet. RL will allow agents to live up to their promise. I will go as far as to say that nearly everything online will soon be controlled by these agents.
That said, I have found it difficult to see the merit in the current crypto agent tokens from an investment perspective. They feel commoditized and rather easy to replicate in current form; I think the underlying models will just have most of these capabilities out of the box per se. If you are building in this space, I think you have to find a way to get IP capture or secure IP sharing revenue.
Preparing for AI-Driven Workflows
Many underestimate the impact AI agents will have on careers. Nearly every industry will integrate agentic workflows, automating entire processes. Many for some reason tend to think their industry is immune, it's not… I'll give you a potentially less traditional example, a mid-sized logistics company implements an AI-agentic workflow to streamline operations and reduce costs. An Order Processing Agent extracts shipment details and assigns deliveries, while a Route Optimization Agent dynamically adjusts routes based on real-time traffic, weather, and fuel prices. A Load Balancing Agent optimizes truck space, and an Exception Handling Agent reroutes shipments when delays occur. Meanwhile, a Customer Update Agent sends automatic notifications, and a Performance Review Agent analyzes fuel efficiency and driver performance. This system could reduce manual decision-making by 90%.
As AI agents take over entire workflows, the nature of work itself will change. The key skill shift, at least in the short to medium term, will be moving from "doing" work to "curating" and overseeing AI-driven processes.
How to Stay Ahead & Profit from This Shift
- • Systematize Your Work – Convert processes into structured workflows
- • Build Modular AI Workflows – Break down tasks into AI-manageable chunks
- • Experiment with AI Orchestration – this may be daunting but clone that github repo and play around with them. No better way to learn
AI agents won't just execute tasks - they'll replace entire roles. You need to learn how to oversee, refine, and leverage them effectively. Do things that allow you to develop specific knowledge. I.e. things that can't be taught and must be learned.
Wearables
Wearable adoption is about to skyrocket. Today, only ~20% of Americans use them (Apple Watch, Whoop, Oura Ring, etc.), but I see that hitting 65-70% by decade's end. Why? Priceless health data and AI-driven personalization.
Right now, billionaires are already using Whoop data to have their meals chosen for them and have a truly tailored health experience that is curated to them. They hire personal assistants and chefs to carry out these services for them. I recently spoke with someone who works with several UHNW individuals, and they described how one client's entire daily routine - from meals to exercise to sleep schedule - is optimized based on continuous biometric feedback.
Soon, these services will be democratized through personalized LLMs. They will also get significantly smaller - I think the optimal size is similar to those balance bracelets half of y'all got psyoped into wearing like 15 years ago.
When humanoid robots become affordable - think $20,000 per unit - everyone will have access to the lifestyle advantages the ultra-rich enjoy today. Gun to my head, you'll begin to start seeing them in 3 years (2028) and at scale in 5-6 but I think the wearable takeoff will accelerate even before this.
In the meantime, get one of these trackers, start collecting your data - your future self will be thankful as I'm convinced this will have a significant impact on your longevity and overall health. The compounding advantage of building your personal health dataset now versus starting in 5 years will be substantial. Get your blood work done at minimum yearly as well.
Market Thoughts
None of this is financial advice, I could completely change my mind on everything tomorrow, I'm not an investment advisor, and this is purely my deranged thoughts written out.
It is becoming increasingly clear that the new administration thinks fiscal austerity is the only answer to dealing with the country's debt and fiscal problems. The richest man in the world outside of maybe Putin and the most powerful person in the world are working hand in hand at this point. I don't particularly believe that the market fully understands this or has in any way priced in a balanced budget.
I wrote a piece on my anonymous account last year discussing how equity markets could remain supported longer than historical averages. My thesis being that the largest companies in the world were deploying record amounts of cash at this idea of digital superintelligence. This felt likely to only increase as the technology continued to improve and the common folk starts to see it integrated into their everyday lives. Being in a cold war with China and the idea of losing that would allow for unprecedented spending in the AI category. Additionally, you had the promise of deregulation and potentially the most pro business president in history likely to be elected - despite what the polls and Karen were telling you.
My point was, don't be so quick to call "top" this go around (as many were last spring and summer). I also talked about the idea of the government actually bidding equities, and it looks as if a national reserve or sovereign wealth fund will start to purchase equities and potentially even cryptocurrencies.
The Longer-Term Outlook
The longer-term impacts of fiscal austerity seem to be less understood. Our country has run and flourished in comparison to the rest of the world under a debt balloon since the 2008 GFC. Our equity markets have been up and to the right in comparison to everywhere else in the world, despite us causing the crisis.
It now appears the people in charge truly believe we are at a tipping point and appear to be taking actions in an attempt to balance the budget. Meanwhile, we still have persistent inflation which is something else that needs to be added to the equation.
Bessent and Trump have been publicly clear that their goal is to bring yields substantially lower even at the expense of equities. Have you noticed how Trump isn't talking about the Dow as he did during his entire first term?
The Potential Market Paradox
The likely impact of this, in my view, will be unprecedented - people will look to the past and get smoked because little is similar, and there isn't a fractal that's meaningful. The rest of the world's a mess; the US has the cleanest clothes in a hamper of dirty laundry.
No one, and I mean no one, is going to turn to Europe. Investors are far too scared of the CCP to entrust dumping significant money into Chinese markets. So you have a paradox where dollars and bonds potentially both move higher while equities and risk assets tumble.
To me, this seems more likely to occur in the next two years than 2027 or 2028, as Republicans have a majority in both chambers of Congress, and you don't want to risk bad economic times going into the Presidential election in 2028. They can scapegoat the previous administration in the short term and have a chance at taking credit for "Saving America." Trump will also want to leave office on a high note as much of what he does revolves around his legacy.
I had thought many of these things through prior to Inauguration but assigned relatively low probabilities to them. Then, the president of the United States, and I would argue the most well-known person in the history of the world outside of Jesus and Hitler, launched a meme coin. Additionally, he has tripled down on DOGE since taking office. These are unprecedented rarities that occur every millennium, that essentially overlapping.
Full disclosure, I am long $TRUMP.
The cryptocurrency action really made me reassess everything. This action made it abundantly clear that he was likely to stick with his extreme campaign promises, cut waste and spending, and really shake up Washington.The president took his own steps to be crypto-denominated, I think few understand the way and the implications of this. But he now has more of his wealth in crypto than dollars, real estate, and every other asset combined.
I'm not here to be Nostradamus and predict a crash, but pain seems likely in the coming 4 quarters. A balanced budget has implications, and I see a sub 5% chance of it being good for equities. In the background, you also have the largest and most famous investor of all time hoarding record amounts of cash and treasuries. Has Warren lost his touch?
The AI Paradox
Shifting gears back to AI, it has been nearly 2.5 years since ChatGPT's public release, neither the utopian promises nor the existential fears of AI have materialized. AI was supposed to revolutionize everything - but computer engineer demand remains high, no industries have been fundamentally disrupted, employment remains strong, and AI-generated content has yet to reshape entertainment. Consultants still charge $500 an hour. The AI revolution always seems to be six months away.
It is clear to me that the market isn't pricing in AI as a force for widespread productivity gains. With gold at ATH and Mega-caps near ATHs, you have to ask: why? This is historically abnormal.
The most logical explanation is that the market expects Mega-caps to consolidate even more power - not because AI is driving broad-based productivity growth, but because it is entrenching attention-based business models. AI will make content and apps more addictive, but it won't boost productivity. In fact, contrary to popular belief (and what these models will likely tell you) we're seeing the opposite. The trend of declining productivity continues, as engagement-driven AI increasingly dominates over efficiency-driven AI. Ads will get smarter, entertainment will get better, and the majority will sink deeper into the attention economy.
This disconnect is striking, given that every billionaire and CEO continues to preach AI's productivity gospel. But if transformative gains were truly imminent, why are gold and Mega-caps both near all-time highs? The market appears to be pricing in something else entirely. Are these leaders selling you a narrative, or are they themselves caught in the hype cycle?
The empirical evidence isn't encouraging. Despite unprecedented access to information, test scores have trended lower for the past decade. Attention spans continue to shrink to new lows. I've searched extensively and found it legitimately difficult to identify any promising trends in education, productivity, or learning ability that correlate with our technological progress.
I don't say this lightly, but the evidence suggests that being short average outcomes and long extraordinary individuals is the rational play. To put this in layman's terms, AI seems unlikely to make the average Joe 30% better but it will provide extraordinary individuals and teams multiple x potential - likely to hit certain domains before others. We're entering an era where the leverage available to exceptional performers is amplified at rates previously unimaginable, while the middle continues to get hollowed out. The probability distribution of success is becoming increasingly bimodal – the bell curve seems likely to flatten in the middle, shift left, and stretch at the far extreme.
I wrote this section on 2/15 - Emini has now shed over 1000 ticks. Bitcoin ~20%. Now 5880 and 80k respectively. If we do start to see a more significant selloff, I think a couple areas are worth watching and will have my attention, 5200 / 4800 on SPX and 69k / 54k on BTC.
How to "Make It" or "Survive" in the AI Era
How do you make it or even just survive in this new paradigm? I haven't "made it" yet, but I'll give you some food for thought…
I get this question a lot, and in all honesty, I resent answering it but I'm going to here so I have something to point people to when they ask me from now on. My brain is wired differently than most or at least I think it is. Everything that I get asked, my brain instantly puts into probabilities and at the same time challenges it, assumes it's wrong, and I tend to have no problem telling people when they're wrong. Not great for maintaining a girlfriend, but rather beneficial for determining how Google's earnings CAPEX announcement is going to impact Broadcom.
That said, I think for me to confidently say you are doing the necessary things to survive and thrive in a world where humans aren't the most intelligent beings, you need to be doing significantly more than just replacing your Google searches with GPT queries.I'll break it down into 3 levels:
Level 1: Understanding Model Nuances
You need to test these models thoroughly so you are aware of the nuances and strengths of different models. I'll give you an example: Models are rather biased based on where they are developed. The models built in California (OpenAI, Meta, Claude, Gemini, Grok) all have their own biases and most have safety guardrails that will influence the decision-making of the models in many instances.
You can compare responses to models like Qwen or Deepseek that are built in China - the differences in outputs can be significant. For certain analytical tasks, you'll find that using multiple models in parallel will bring you a more comprehensive understanding than relying on any single one. Personally, I think knowing these nuances is the bottom of the barrel and something you absolutely need to understand. I think this puts you ahead of 80% of people, and you can probably keep your email job for the time being.
Level 2: Building AI-Enhanced Workflows
At this level, you're not just using AI tools - you're deliberately designing systems where AI augments your thinking process. This means creating structured workflows where AI handles specific cognitive tasks while you focus on higher-order thinking.
I'll give you some examples:
-
• Setting up automated research pipelines where AI continuously monitors, filters, and summarizes information in your field
-
• Developing personal frameworks for when to delegate thinking to AI versus when to rely on your own judgment
-
• Think creating "second brain" systems where AI helps organize, retrieve, and connect your knowledge
The key difference between Level 1 and Level 2 is that you're no longer just using AI tools - you're creating systems where AI becomes an extension of your thinking process. You're building infrastructure that amplifies your capabilities rather than simply outsourcing discrete tasks.
Here's an example from my life:
When evaluating an investment opportunity, I have a structured workflow where different models analyze different aspects - one evaluates market trends, another scrutinizes financials, and a third plays devil's advocate. I then integrate these perspectives into my decision-making framework. Your probability of "making it" I tend to believe increases rather drastically if you can get yourself to the far spectrum of this level.
Level 3: Seamless AI Integration
At this level, the boundary between your thinking and AI assistance becomes nearly invisible. AI is woven into the fabric of your daily cognition and decision-making in ways that feel natural and frictionless.
Here are two examples that illustrate the leap from Level 2 to Level 3:
-
Communication Enhancement: Someone texts asking if you're free for drinks next Thursday, instead of reading the message, checking your calendar, and then going back to reply - your AI-integrated system automatically overlays calendar information alongside the message with personalized response options based on your past communication patterns. You're not thinking "let me check with my AI" - it's just part of how you process information now.
-
Research: When researching a complex topic, you no longer manually prompt different AI systems. Instead, your integrated environment continuously pulls relevant information from various sources, organizes it according to your unique mental models, identifies gaps in your understanding, and generates connections - all while adapting to your evolving thought process in real-time.
The key difference between Level 2 and Level 3 is that Level 2 still requires conscious engagement with AI systems—you're aware of using tools and orchestrating workflows. In Level 3, AI becomes an unconscious extension of your thinking.
I personally am at Level 2 but think that Nvidia Digits - which I discussed previously will enable the transition to early stages of Level 3.
Cognitive Flexibility
In this rapidly evolving landscape, the most valuable skill isn't any specific technical knowledge - it's cognitive flexibility. This goes beyond mere adaptability, it's about fundamentally rewiring how you think. What separates those who will thrive from those who merely survive is the ability to:
-
• Recognize when your biological intelligence should lead and when AI should lead and seamlessly alternate between these modes based on the nature of the problem.
-
• Deliberately expose yourself to AI outputs that challenge your thinking and biases, using this friction as a catalyst for personal development.
-
• Constantly question how you know what you know, and use AI not just as a tool but as a mirror that reflects your own thinking limitations and blindspots.
-
• Have an explorative mindset, be willing to experiment with new tools and approaches, and most importantly, developing your own frameworks for evaluating what works and what doesn't - these are the true differentiators.
I don't think it is possible to be an expert in every AI tool or technique, and I think it is -ev even trying. The goal is to develop an intuitive understanding of how these systems think and how you can most effectively collaborate with them. This is less about technical mastery and more about developing a new form of literacy - one that enables a fundamentally different kind of human cognition.
Looking Ahead
As I wrap up this first post, I want to emphasize that we're living through what is likely to be the most significant technological transition in human history. The next few years will bring many challenges and an abundance of opportunities, we're living in exciting times - lock in.
You're gonna make - say it back.
TG